Utility companies face several challenges such as energy cost volatility, supply-chain disruptions, increasing customer demands for decarbonization and clean energy, and the need for personalized experiences. AI adoption can help them to streamline operations, optimize resource management, enhance customer interactions, and develop new digital services.
Learn the benefits of AI utilities, and how they help utilities via use cases and real-life examples:
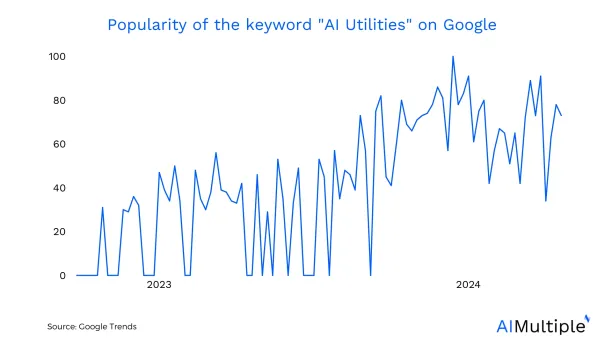
AI utilities use cases & real-life examples
Energy
1. Autonomous operations in power plants
AI automates plant inspections by analyzing data from cameras and sensors in real time, reducing reliance on human workers and enhancing safety by detecting leaks or other hazards promptly. This automation meets the demands of an aging workforce and enhances plant efficiency.
Real-life example:
Duke Energy, aiming to achieve net-zero methane emissions by 2030, faced challenges in monitoring natural gas pipelines for leaks. They partnered with Microsoft and Accenture to develop a new platform using Microsoft Azure and Dynamics 365 to integrate satellite, ground sensor data, and AI for real-time leak detection and response.
The platform assessed emissions data, prioritized repair areas, and dispatched crews promptly, helping to reduce greenhouse gas emissions.
- Provided graphic dashboards for prioritizing leak repairs
- Enabled precise geolocation data for quicker repairs
- Scalable to other emission sources and equipment.
2. Energy demand forecasting
Efficient utility distribution relies on accurately forecasting energy and water demand, which constitutes a major portion of operational costs. AI in energy demand forecasting helps utility companies manage supply and demand by analyzing factors such as weather patterns, user behavior, and market prices by:
- Forecasting energy demand and optimizes supply distribution
- Predicting renewable energy availability and balances with other sources
- Enabling price optimization based on historical data and potential competitor responses
- Encouraging efficient consumer behavior by notifying users about peak periods.
This predictive capability leads to reduced operational expenses, optimized equipment runtimes, better scheduling and resource management, and ensures a balanced supply-demand equation, promoting sustainability. This is especially helpful when integrating renewable energy sources like solar or wind, which are weather-dependent.
Real-life example:
AES, transitioning from fossil fuels to renewables, needed predictive tools for energy output, maintenance, and load distribution. Collaborating with H2O.ai, AES deployed predictive maintenance programs for wind turbines, smart meters, and optimized its hydroelectric bidding strategies.
The platform enabled AES to anticipate component failures, optimize repair costs, and manage demand prediction, helping the company reduce costs and increase reliability.
- Saved $1 million annually by reducing unnecessary repairs
- Achieved a 10% reduction in customer outages
- Addressed 85 operational challenges over two years.
3. Energy Prosuming
AI solutions for energy prosumers help users manage self-produced energy from sources like solar panels or wind turbines. These solutions optimize the use of renewable energy and enable users to sell surplus power back to the grid.
- Balances supply and demand based on consumption peaks and weather conditions.
- Integrates with smart meters for efficient energy management.
- Supports surplus energy trading or sharing with the local grid.
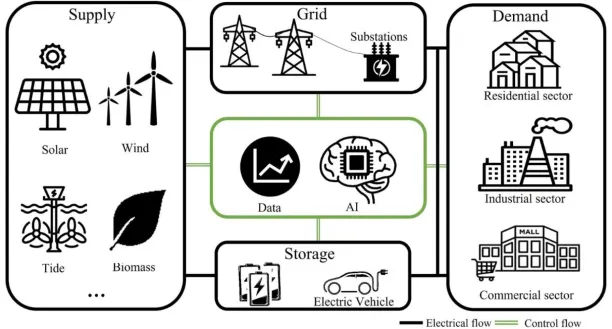
4. Industrial digital twins for power generation
AI-driven digital twins create virtual replicas of power generation sites like wind turbines, allowing utilities to simulate and predict maintenance needs, optimize performance, and reduce downtime. These models can accurately forecast issues like corrosion, minimizing disruptions and increasing reliability in power supply.
Real-life example:
For instance, Google’s neural network improved wind energy forecast accuracy, boosting financial returns by 20%. This predictive capability allows for efficient scheduling of energy production and consumption, maximizing resource utilization and profitability.
Real-life example:
Siemens Energy’s digital twin for heat recovery steam generators predicts corrosion, potentially saving utilities $1.7 billion annually by reducing inspection needs and downtime by 10%. Siemens Gamesa’s digital twin simulates offshore wind farm operations 4,000 times faster, optimizing turbine layouts and cutting energy costs.
5. Power grid simulation
AI-driven grid simulations allow utilities to model power flow, schedule outages, and test grid resilience, especially with the increased integration of renewable energy sources. This optimizes maintenance and outage management, ensuring minimal impact on customers.
6. Smart Homes as energy hubs
AI-based smart home systems help homeowners monitor and adjust energy usage, reducing costs and minimizing demand on the grid through better load management.
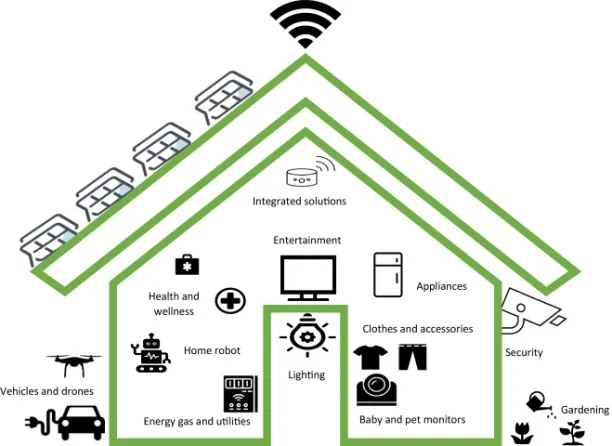
7. Smart meters for real-time power flow
AI-driven smart meters integrate with distributed energy resources to balance demand and supply in real-time, supporting grid resilience and decarbonization efforts.
Real-life example:
Con Edison, a utility company, aimed to reduce operational costs and environmental impact by leveraging artificial intelligence. AI-powered tools helped lower power generation costs and reduce CO₂ emissions, empowering customers with more control over energy usage.
This AI-driven approach not only streamlined operations but also supported Con Edison’s commitment to sustainability and customer-focused energy solutions.
- Reduced power generation costs and CO₂ emissions
- Enabled enhanced customer energy management
- Promoted eco-friendly and customer-centric operations.
Waste
8. Waste management
AI in waste management aids in tracking, analyzing, and optimizing waste disposal and recycling processes. It collects data on waste types, volumes, and patterns, allowing for better resource management and waste reduction.
- Tracks and analyzes waste patterns to inform pick-up schedules.
- Predicts future waste levels for improved planning.
- Identifies and sorts recyclable materials with computer vision and machine learning.
- Reduces food waste by identifying discarded food types and quantities.
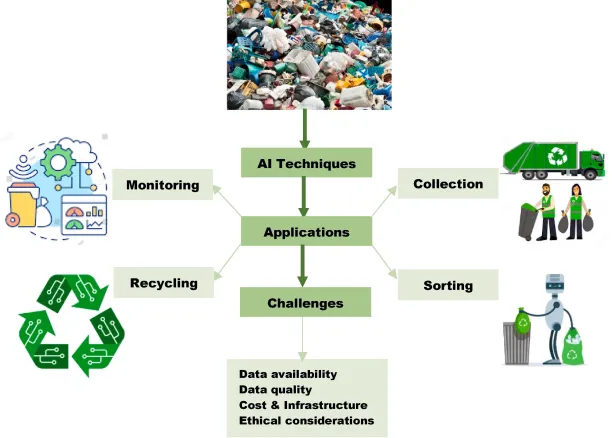
Water
9.Water quality monitoring
AI can enhance water quality monitoring by analyzing water flow and detecting contaminants in real time. AI-enabled sensors deployed in water systems identify harmful bacteria and particles, enabling faster responses to potential health risks.
- Monitors water quality continuously, detecting contaminants in real time.
- Improves transparency and control over water supply systems.
- Supports quick actions in response to health risks.
Real-life example
Fluid Analytics uses AI-powered software, robotics, and IoT to optimize urban water systems with predictive models trained on varied pipeline data. Cities, especially in India, sought their help to locate leaks, reduce water loss, and prevent flooding due to outdated infrastructure and inspection methods. Fluid Analytics’ results include:
- Monitoring over 400 million gallons of urban wastewater daily
- Mapping drainage channels to prevent severe flooding near Mumbai airport
- Facilitating early detection of waterborne diseases and preventing outbreaks, such as hepatitis-A.
Industry-agnostic use cases
10. Automated asset maintenance
Energy and utilities companies struggle to detect defects in critical infrastructure, leading to costly breakages. AI analyzes aerial imagery, LiDAR, drone and satellite data to identify equipment issues or vegetation risks that could damage infrastructure.
For instance, AI-powered image recognition and computer vision can analyze drone-captured images of assets, allowing for rapid identification of potential failures. This proactive monitoring minimizes service disruptions and reduces fire hazards around power lines, eventually optimizing resource scheduling.
Real-life example:
Exelon, a large energy company, sought to improve its grid maintenance and inspection process. Using NVIDIA’s AI tools for drone inspections, Exelon enhanced its defect detection capabilities, creating labeled examples for real-time assessment.
This AI-driven approach improved maintenance accuracy, minimized emissions, and increased the reliability of the energy grid.
- Enhanced grid defect detection through AI-driven drone inspections
- Increased maintenance efficiency and grid reliability
- Reduced emissions through optimized inspection processes.
11. Automated customer service experience
Utility suppliers can enhance customer engagement by predicting water and energy consumption with AI, allowing for dynamic pricing strategies. By analyzing usage patterns, AI can suggest optimal usage times for cost savings, such as recommending later charging times for electric vehicles. This personalized approach improves customer satisfaction and supports targeted marketing efforts, ultimately increasing loyalty and revenue.
Real-life example:
Octopus Energy, an energy provider, sought to improve its customer service through enhanced email response quality. They implemented Generative AI to automate responses to customer emails, achieving an 80% customer satisfaction rate, surpassing the 65% rate of human agents.
By using Generative AI, Octopus Energy streamlined its customer support process, ensuring quick and accurate responses, demonstrating AI’s potential in the utilities sector.
- Achieved 80% customer satisfaction in AI-driven email responses
- Outperformed trained human staff’s satisfaction score by 15%
- Showcased potential for further AI integration to improve customer loyalty.
12. Fleet optimization for utility trucks
The energy sector’s complex supply chains require efficient logistics management. AI enhances coordination between operations teams and warehouses, optimizing fleet management and route planning.
For instance, AI optimizes utility truck routes during outages and extreme weather, reducing travel times and improving response times to restore services more quickly. This leads to improved delivery times, reduced operational costs, and better alignment with market demand.
13. Substation safety and security
AI-based video analytics improve substation security by detecting unauthorized intrusions and monitoring worker safety, enhancing compliance and reducing potential incidents.
14. Virtual assistants in call centers
Improvement: AI virtual assistants support customer service by managing call surges, assisting with FAQs, and providing usage insights, which improves customer experience and reduces operating costs.
Real-life example:
Ontario Power Generation (OPG), a major Canadian electricity producer, aimed to improve internal efficiency and support for its employees. In collaboration with Microsoft, OPG developed ChatOPG, an AI-powered virtual assistant that answers queries, provides information, and acts as a personal assistant.
The chatbot supports productivity, enhances safety, and streamlines performance by offering workers easy access to needed information.
- Improved employee productivity and access to information
- Enhanced safety and operational efficiency
- Promoted AI integration in daily operations for better performance.
Telecom
15. Network operations
Zero-Touch Network Operations
Zero-touch network operations involve using AI to automate network management tasks, reducing the need for human intervention. This includes self-monitoring, self-healing, and automatic optimization of network resources. By integrating digital twins and machine learning, telecom operators can achieve higher service reliability and operational efficiency.
Real-life examples: Ericsson implemented AI-driven zero-touch operations, leveraging machine learning and digital twins for autonomous management. This enhanced service reliability and reduced manual tasks, boosting operational efficiency. As a result, Ericsson could
- Enable autonomous operation with minimal oversight
- Increase network reliability
- Improve service efficiency.
Network Optimization and Management
AI-driven network optimization involves using predictive analytics to monitor and enhance network performance in real-time. This ensures that the network remains efficient, reducing downtime and enhancing user experience. The system analyzes large volumes of data to predict and address potential issues before they impact services.
Real-life example: Nokia’s AVA platform used AI-based predictive analytics for real-time network management, optimizing performance and minimizing service disruptions. This way,
- Enhanced real-time network performance
- Reduced downtime
- Improved user satisfaction.
5G Network Slicing
AI supports 5G network slicing by enabling network function virtualization. This allows telecom operators to create and allocate network segments dynamically for different use cases and customer needs, which increases efficiency and opens up new revenue opportunities.
Real-life example: Huawei used AI to support 5G network slicing, dynamically allocating resources to provide tailored services and maximize network utility. This way, Huawei could achieved:
- Tailored services for different use cases
- Improved resource management
- New revenue opportunities.
Data Traffic Management
AI-powered data traffic management optimizes the allocation of network bandwidth based on real-time demand. This ensures that during peak times, network performance is maintained, leading to a better user experience and more efficient use of resources.
Real-life examples: Ericsson’s AI solution optimized data traffic management by adjusting bandwidth allocation in real-time, ensuring consistent network performance. This way,
- Optimized bandwidth usage
- Stable network performance during peak times
- Enhanced service quality.
Why should we use AI in utilities?
Using AI in utilities can help address the surging demand for electricity driven by data centers and electric vehicles, and unlock investment opportunities, as some utility trends suggest. Here’s how:
- Electricity demand surge: Electricity demand is projected to increase 1.4% annually through 2032, driven by data centers and electric vehicles, leading to a 46% cumulative rise. As a result, utilities will face increased pressure to meet this growing demand to generate electricity without disrupting supply.
- AI-powered demand forecasting can provide utilities with precise demand predictions, enabling efficient energy allocation and proactive planning to prevent grid overload. AI-powered scheduling can lead to a 25% to 30% improvement in field productivity, helping utilities manage resources more effectively.
- Investment opportunities in utilities: Analysts see an investment opportunity, as utility stocks are currently undervalued by 5% and don’t fully account for upcoming data center demand. This undervaluation presents potential for investors as demand from data centers grows.
- AI analytics can identify trends in demand and pricing, helping investors and utilities strategically invest in capacity expansion to capitalize on this growth. AI utilities can reallocate up to 80% of capital based on asset health using machine learning insights.
- Data center demand growth: Data center electricity demand could double by 2030, with a bull-case scenario predicting a 131% increase by 2032. As data centers become major energy consumers, utilities must enhance capacity and efficiency to handle demand spikes.
- AI can optimize data center energy use through predictive analytics, reducing consumption without compromising performance, easing grid pressure and improving sustainability. For example, AI helped increase the heat rate or yield for fossil and renewable generation assets by 2% to 5%.
What are AI utilities?
AI utilities refer to AI use in utility industry by using machine learning (ML) and generative AI, to enhance efficiency and operations. This technology leverages real-time data, predictions, and automation to help companies optimize processes across customer service, maintenance, and system management.
Solutions under AI utilities
Energy companies can benefit from these cutting edge technology advances:
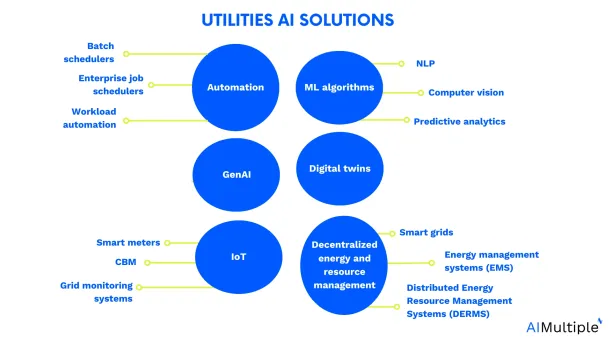
Automation
These tools can automate routine tasks such as meter reading and billing processes, reducing operational costs and minimizing human error in data management.
- Workload Automation: Workload automation solutions streamline and manage repetitive tasks across various systems, enabling utilities to increase operational efficiency and reduce manual errors while ensuring critical processes run smoothly.
- Batch Scheduling: Batch scheduling software organizes and executes large volumes of tasks or processes in groups at scheduled times, allowing utilities to optimize resource allocation and ensure timely completion of jobs without disrupting ongoing operations.
- Enterprise Job Scheduling:Enterprise job scheduling software coordinates and prioritizes tasks across an organization’s IT landscape, helping utilities improve service delivery, enhance system utilization, and maintain consistent performance by ensuring that jobs are executed in the correct order and on time.
Machine learning algorithms
These algorithms enhance decision-making by identifying patterns in consumption data, facilitating demand-side management strategies and personalized energy solutions for consumers. Here are some of these tools:
- Natural Language Processing (NLP): NLP can improve customer service chatbots and virtual assistants, providing instant support and improving customer engagement by understanding and responding to inquiries in real time.
- Computer Vision: Computer vision leverages image analysis from drones and cameras to inspect infrastructure, enabling faster and safer identification of equipment issues compared to manual inspections.
- Predictive analytics: Predictive analytics tools utilises historical data to forecast demand and detect potential failures in infrastructure, allowing utilities to preemptively address issues and optimize resource allocation.
Internet of Things (IoT)
IoT devices and sensors for real-time monitoring of grid performance and energy consumption, enabling proactive maintenance and improved grid reliability. Some examples include:
- Smart meters: Smart meter solutions provide real-time data on energy consumption, enabling accurate billing and efficient energy management.
- Real-time monitoring systems for grid reliability: These systems track grid performance continuously, allowing utilities to detect issues early and maintain reliable service.
- Condition-based maintenance (CBM): CBM monitors equipment health to schedule maintenance only when needed, reducing costs and preventing unexpected failures.
Generative AI
Generative AI uses advanced algorithms and machine learning to create predictive models and simulations from historical data and various scenarios. In the utility sector, this technology optimizes energy distribution and improves forecasting accuracy. For example, generative AI helps with:
- Renewable energy integration to evaluate how to incorporate renewable energy sources by simulating their impact on overall grid stability and reliability.
- Asset management by allowing utilities to schedule repairs or upgrades based on projected performance and risk factors.
Digital twins
Digital twins create virtual models of physical assets, allowing utilities to simulate and analyze performance under various scenarios, leading to better asset management and operational efficiency. By processing various data sources, these models enhance operational efficiencies and compliance with environmental standards.
Implementing AI-driven digital twins can result in significant energy savings and carbon footprint reductions, supporting sustainability goals.
Decentralized energy and resource management
These tools enhance the management and integration of renewable energy sources, promoting resilience and flexibility. Some of them include
- Smart Grids: Smart grid solutions analyze real-time data to balance energy flow and integrate renewables. Leverages AI to analyze data from connected devices, facilitating real-time adjustments to energy flow, improving grid resilience, and enhancing integration of renewable energy sources.
- Distributed Energy Resource Management Systems (DERMS): These systems can manage decentralized resources like solar and battery storage. Coordinates the management of decentralized energy resources like solar and batteries, optimizing their contribution to the grid while ensuring reliability.
- Energy Management Systems (EMS): EMS can integrate AI algorithms to optimize energy production, storage, and consumption, leading to more efficient operations and reduced costs.
Benefits of AI in utilities industry
AI helps utility companies to:
- Simplifying complexity: AI can simplify intricate workflows within the energy and utilities sector by using AI assistants to optimise processes, simulate operations, diagnose real-time issues, ensure supply chain traceability, and provide immediate technical support. This leads to increased efficiency, reduced costs, and minimized downtime.
- Driving cost and energy efficiency: Generative AI solutions enhance energy efficiency and significant cost savings by offering a holistic view of operations. This allows power companies to accurately measure emissions and optimize processes, thereby accelerating the energy transition and promoting sustainability and operational excellence.
- Scaling innovation: Collaborations like those with AWS leverage a vast partner network and industry expertise to rapidly adopt advanced technologies, including generative AI. This helps utility companies scale innovative clean energy technologies efficiently, allowing them to meet energy demands while facilitating the sector’s transition to cleaner practices.
- Generating data-driven strategy: AI assists with data strategy, helping utilities make risk-based replacement and maintenance decisions by analyzing customer risk, safety, and environmental factors. For instance, generative AI combined with ML can process images and videos to identify defects in supply lines, reducing maintenance costs and maintaining reliability.
- Ensuring maintenance: Generative AI combined with ML improves maintenance by detecting and predicting equipment issues. It offers interactive troubleshooting, helping field workers quickly resolve technical issues.
AI utilities challenges
Here are some challenges of adopting AI in utility industry:
- Data privacy: Training AI systems requires large amounts of data, raising concerns about customer data privacy. While there’s potential to optimize this data to better understand customer needs, ensuring privacy protection remains a significant challenge.
- AI bias: AI systems can exhibit biases, which may lead to unfair treatment of customers or employees. Human oversight is necessary to address AI biases and ensure that AI implementation meets ethical standards. Although training systems can reduce bias, it may not eliminate it entirely, making human supervision crucial.
Discover other AI limitations and challenges.
Further reading
Explore more on AI in other industries: