This did not happen because the robot was programmed to do harm. It was because the robot was overly confident that the boy’s finger was a chess piece.
The incident is a classic example of something Sharon Li, 32, wants to prevent. Li, an assistant professor at the University of Wisconsin, Madison, is a pioneer in an AI safety feature called out-of-distribution (OOD) detection. This feature, she says, helps AI models determine when they should abstain from action if faced with something they weren’t trained on.
Li developed one of the first algorithms on out-of-distribution detection for deep neural networks. Google has since set up a dedicated team to integrate OOD detection into its products. Last year, Li’s theoretical analysis of OOD detection was chosen from over 10,000 submissions as an outstanding paper by NeurIPS, one of the most prestigious AI conferences.
We’re currently in an AI gold rush, and tech companies are racing to release their AI models. But most of today’s models are trained to identify specific things and often fail when they encounter the unfamiliar scenarios typical of the messy, unpredictable real world. Their inability to reliably understand what they “know” and what they don’t “know” is the weakness behind many AI disasters.
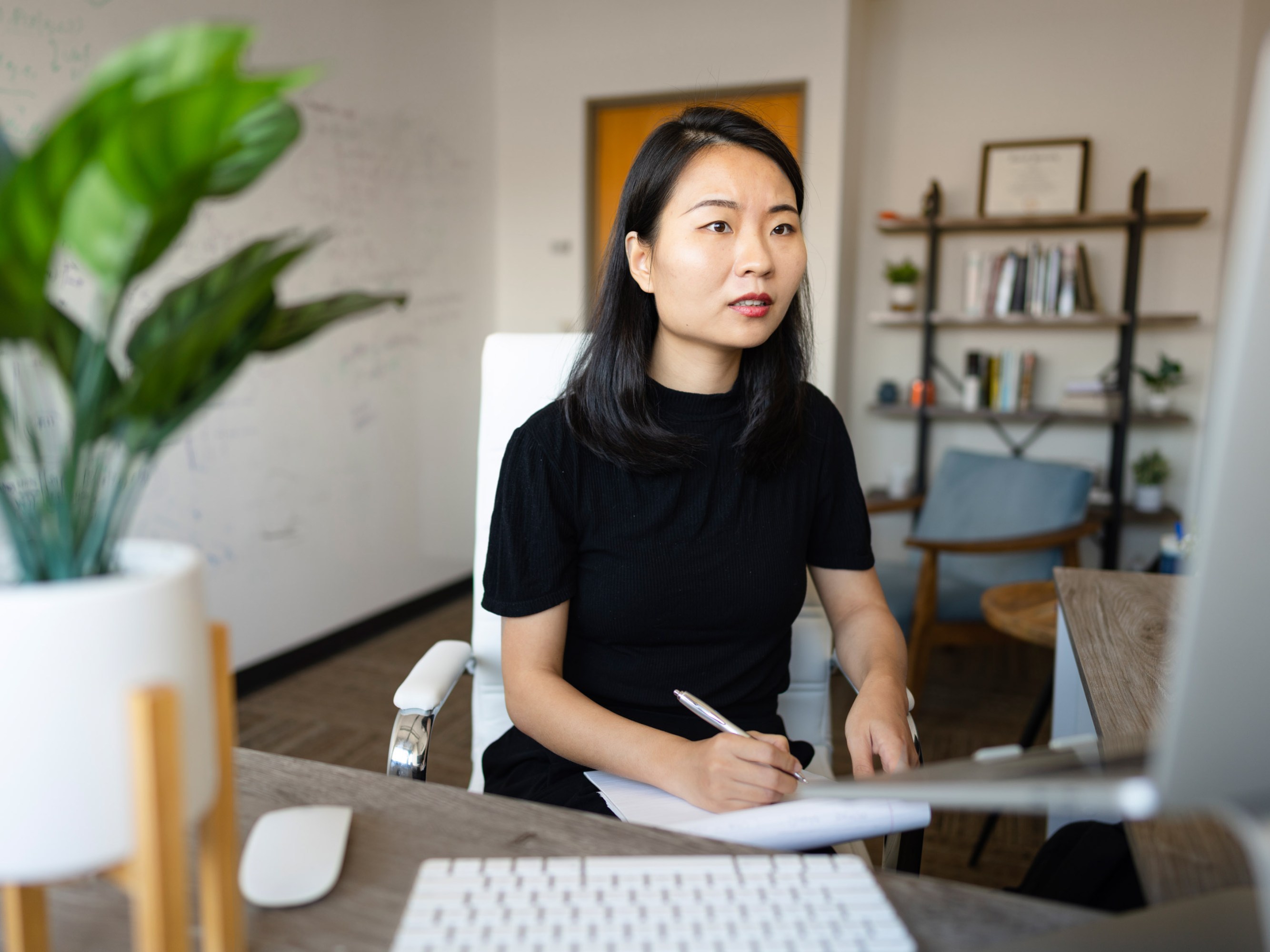
SARA STATHAS
Li’s work calls on the AI community to rethink its approach to training. “A lot of the classic approaches that have been in place over the last 50 years are actually safety unaware,” she says.
Her approach embraces uncertainty by using machine learning to detect unknown data out in the world and design AI models to adjust to it on the fly. Out-of-distribution detection could help prevent accidents when autonomous cars run into unfamiliar objects on the road, or make medical AI systems more useful in finding a new disease.